流石に翻訳はないか。
https://waymo.com/open/
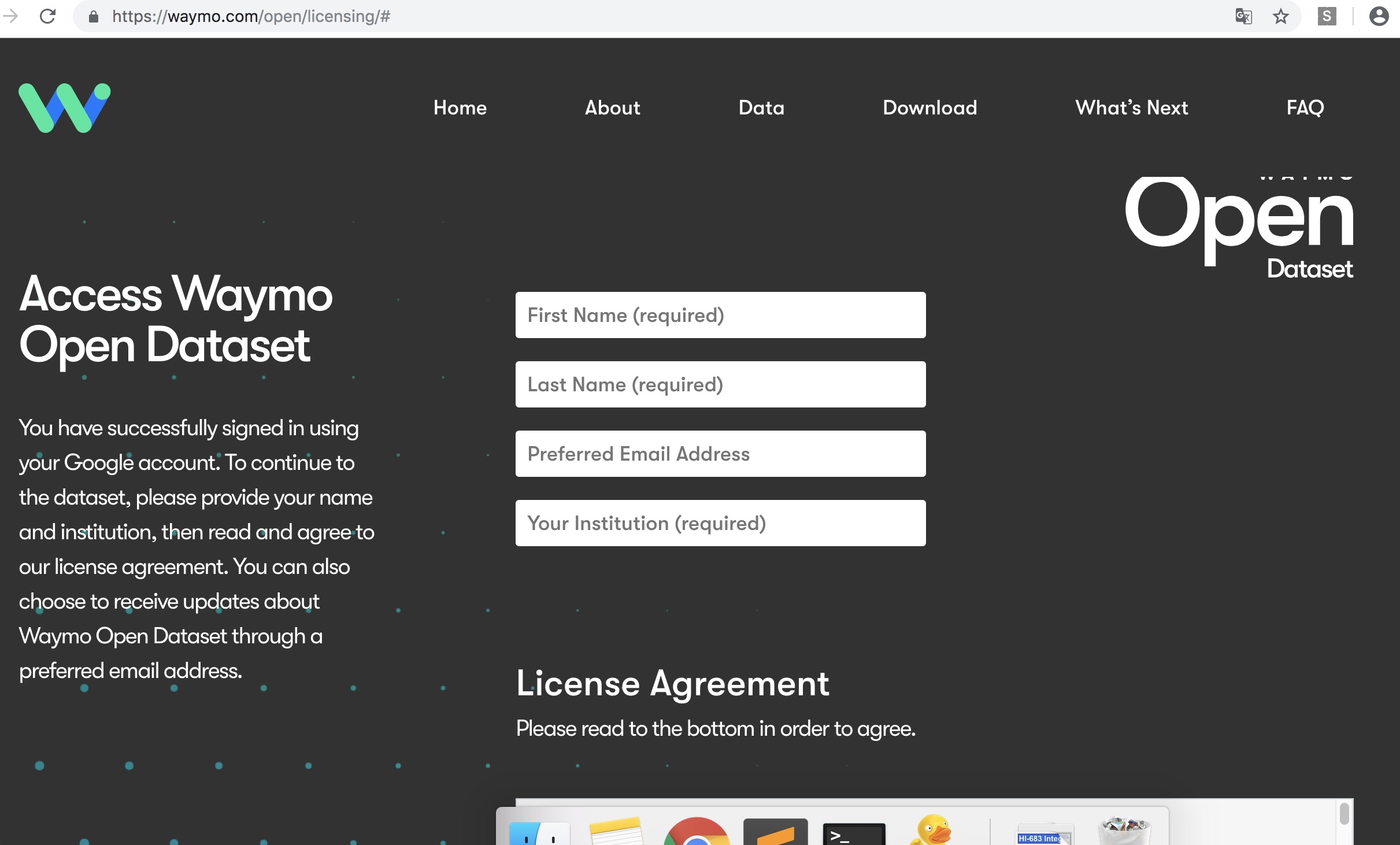
>The Dataset is about 1TB after compression for 1000 segments. Segments have been packaged into multiple files of about 25GB each.
>All segments contain 3D labels. The 100 segments that also contain 2D labels have been packaged into the first three files under ‘Training’ and the first file under ‘Validation’.
何これ?git hubもありますね。pythonです。
https://github.com/waymo-research/waymo-open-dataset/blob/master/tutorial/tutorial.ipynb
google colab
https://colab.research.google.com/notebooks/welcome.ipynb#recent=true
[vagrant@localhost local]$ python -V
Python 3.5.2
system requirement
– g++ 6 or higher.
– Python 3.
– TensorFlow 1.14.0 or higher.
g++って、c++じゃん。使わんなー
とりあえずgit clone
[vagrant@localhost waymo]$ git clone https://github.com/waymo-research/waymo-open-dataset.git waymo-od
Initialized empty Git repository in /home/vagrant/local/waymo/waymo-od/.git/
remote: Enumerating objects: 50, done.
remote: Counting objects: 100% (50/50), done.
remote: Compressing objects: 100% (38/38), done.
remote: Total 174 (delta 17), reused 35 (delta 12), pack-reused 124
Receiving objects: 100% (174/174), 14.00 MiB | 2.93 MiB/s, done.
Resolving deltas: 100% (45/45), done.
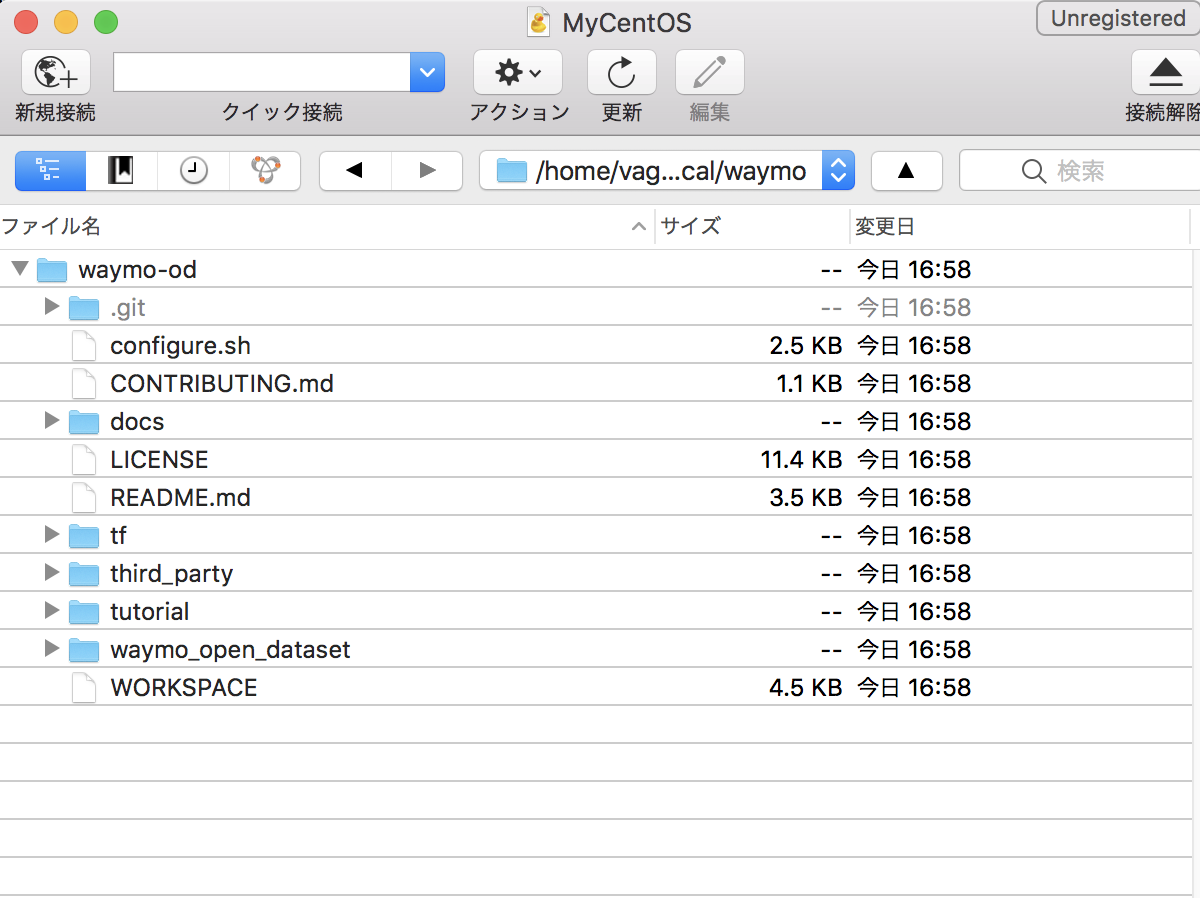
[vagrant@localhost waymo-od]$ git checkout remotes/origin/r1.0
Note: checking out ‘remotes/origin/r1.0’.
You are in ‘detached HEAD’ state. You can look around, make experimental
changes and commit them, and you can discard any commits you make in this
state without impacting any branches by performing another checkout.
If you want to create a new branch to retain commits you create, you may
do so (now or later) by using -b with the checkout command again. Example:
git checkout -b new_branch_name
HEAD is now at 2f30db6… Merge branch ‘master’ into r1.0
[vagrant@localhost waymo-od]$ git branch
* (no branch)
master
あれ?
sudo apt-get install --assume-yes pkg-config zip g++ zlib1g-dev unzip python3 python3-pip
BAZEL_VERSION=0.28.0
wget https://github.com/bazelbuild/bazel/releases/download/0.28.0/bazel-${BAZEL_VERSION}-installer-linux-x86_64.sh
sudo bash bazel-${BAZEL_VERSION}-installer-linux-x86_64.sh
sudo apt install build-essential
これ、debian向けに書かれてる?
そして、bazelってビルドツール?makeでないんかい。